Table of Contents
Introduction
Did you know the global natural language processing market is set to hit $36.4 billion by 2026? This huge growth is thanks to new AI tech like Retrieval-Augmented Generation (RAG). It’s changing how we get and use information.
RAG is a top AI method that mixes language models with knowledge retrieval. This combo makes answers more accurate and relevant. It’s used in many areas, from answering questions to creating chatbots and writing text. RAG is set to change what we think AI can do.
Key Takeaways
- Retrieval-Augmented Generation is a pioneering AI method that combines language models and knowledge retrieval.
- Retrieval-Augmented Generation allows for more accurate and contextual responses, transforming sectors such as open-domain question answering and conversational AI.
- RAG’s ability to use both language models and external knowledge sources makes it an effective tool for text production and data processing.
- The global natural language processing market is estimated to reach $36.4 billion by 2026, with Retrieval-Augmented Generation playing an important role in this expansion.
- Understanding the principles of Retrieval-Augmented Generation is critical for keeping up in the quickly changing world of AI-powered information access and processing.
What is RAG?
Retrieval-Augmented Generation is a new AI method. It combines language models with outside knowledge. This way, Retrieval-Augmented Generation gives better answers by using language models and knowledge retrieval.
Retrieval-Augmented Generation works by adding info from databases to language models. This lets the model use the right data. So, it gives answers that are both accurate and clear. This makes rag and retrieval-augmented generation very useful for many tasks.
Demystifying Retrieval-Augmented Generation
RAG is a big step forward in NLP. It mixes language models with knowledge search. This makes RAG better at solving hard tasks.
With RAG, language models can understand and create text better. They can use info from outside sources. This is great for tasks like answering questions and chatting.
- Retrieval-Augmented Generation makes language models smarter by giving them lots of knowledge.
- The mix of language models and knowledge retrieval helps RAG give better answers.
- Retrieval-Augmented Generation is great for tasks that need to understand lots of context, like answering questions and chatting.
Using rag and retrieval-augmented generation opens up new areas in AI. It helps with getting and understanding information better.
The Power of Language Models and Knowledge Retrieval
In the field of artificial intelligence, integrating language models and knowledge search is an important advancement. Large language models (LLMs) have changed how we process natural language. Yet they are not always able to use information from other sources. This is where Retrieval-Augmented Generation comes in, which connects language models with information.
Retrieval-Augmented Generation uses the strengths of language models and adds in outside knowledge. This makes AI systems give better, more informed answers. It’s a new way to use natural language processing, information extraction, and making decisions based on knowledge.
At the core of Retrieval-Augmented Generation is the idea that language models can’t always know everything. They can create smooth text but lack deep knowledge. Retrieval-Augmented Generation lets them tap into a vast amount of information. This way, they can give more detailed and accurate answers.
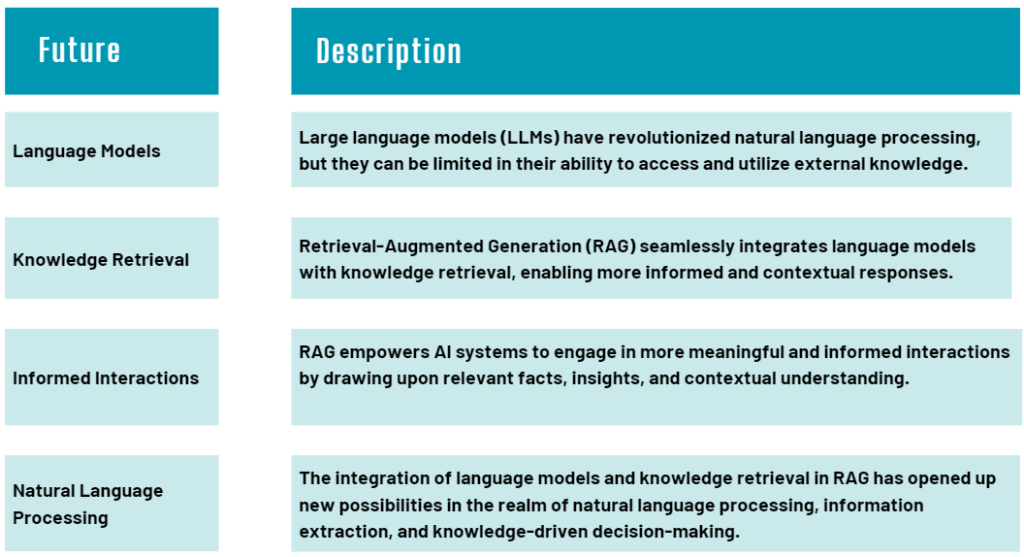
The future of artificial intelligence will be shaped by the power of language models and knowledge retrieval. RAG is a key part of this, helping with information access, knowledge management, and smart decision-making.
Open-Domain Question Answering with RAG
Retrieval-Augmented Generation (RAG) is changing how we talk to AI. It combines language models with knowledge retrieval. This lets AI chat in a more natural and helpful way.
Enabling Conversational AI Assistants
RAG can use a huge knowledge base to answer many questions. This is a big step for AI assistants. They can now understand and answer complex questions better than before.
RAG’s AI can quickly find and mix information from different places. It gives answers that are right and fit the user’s question. This makes conversations more useful and interesting.
“RAG’s open-domain question answering capabilities are the foundation for building conversational AI assistants that can understand natural language and provide meaningful, context-aware responses.”
RAG mixes language models and knowledge retrieval. This lets AI handle all kinds of questions. It makes talking to AI feel more natural and easy.
RAG is making AI assistants more important for getting information. They can tackle tough questions and give answers that fit the situation. RAG is a big step towards making AI talk like we do.
Natural Language Processing: The Backbone of RAG.
Natural language processing (NLP) is the foundation of retrieval-augmented generation . It brings together language models, information extraction, and text generation. This combination enables AI systems to understand and interpret human language quite effectively.
RAG use powerful NLP techniques to evaluate massive amounts of unstructured data. It extracts valuable ideas and knowledge. This information is then utilized in language models. This allows Retrieval-Augmented Generation to generate fluent and fact-based responses.
Text generation is an important aspect of Retrieval-Augmented Generation. It employs high-quality language models to generate content that sounds like it was authored by a person. This text is specific to the user’s requirements and context. It’s ideal for chatbots, question-and-answer systems, and more.
Information Extraction and Text Generation
Retrieval-Augmented Generation is a new way to use language models and knowledge retrieval together. It’s great at pulling out important info from big, messy data and making text that’s both useful and interesting. This method is changing how we deal with unstructured data in big ways.
At its core, RAG mixes language models and knowledge retrieval. Language models learn from huge amounts of text and can write in a way that sounds real. When they work with finding the right info, Retrieval-Augmented Generation opens up new areas in AI for processing and making content.
Extracting Insights from Unstructured Data
Unstructured data, like articles and social media, is full of useful info. Retrieval-Augmented Generation can dig through this data and find the most important parts. It uses its deep understanding of language and info search skills to turn messy data into useful insights. This helps businesses and researchers make better choices.
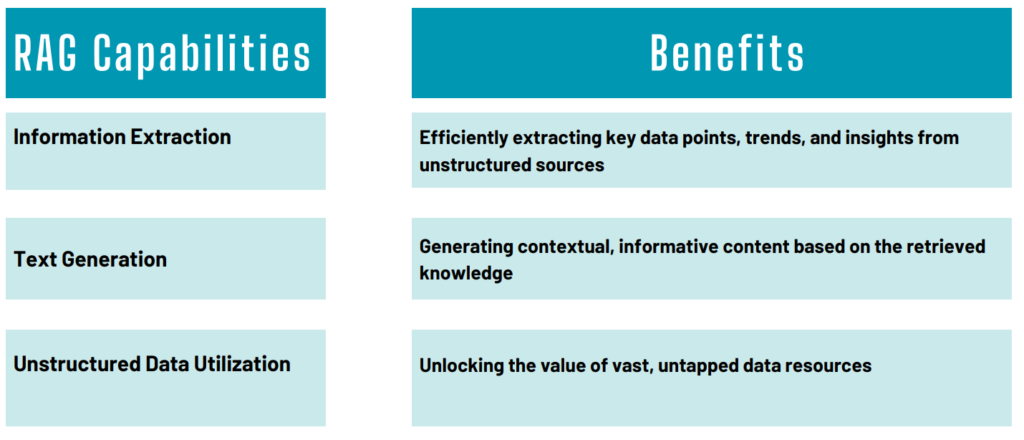
Machine Learning Meets Human Knowledge.
In the area of artificial intelligence, machine learning and human expertise combine in a powerful way. This is known as Retrieval-Augmented Generation. It employs language models to access massive volumes of data. This helps to close the gap between artificial and human intelligence.
RAG is a mix of machine learning’s power and human knowledge’s depth. It combines language models with knowledge retrieval. This way, RAG systems can have more informed and detailed conversations. It opens up new possibilities for AI.
At the core of Retrieval-Augmented Generationis the blend of machine learning and human knowledge. Language models, trained on huge datasets, can understand and create natural language. With knowledge retrieval, they can find and use the right information. This makes their responses more accurate and helpful.
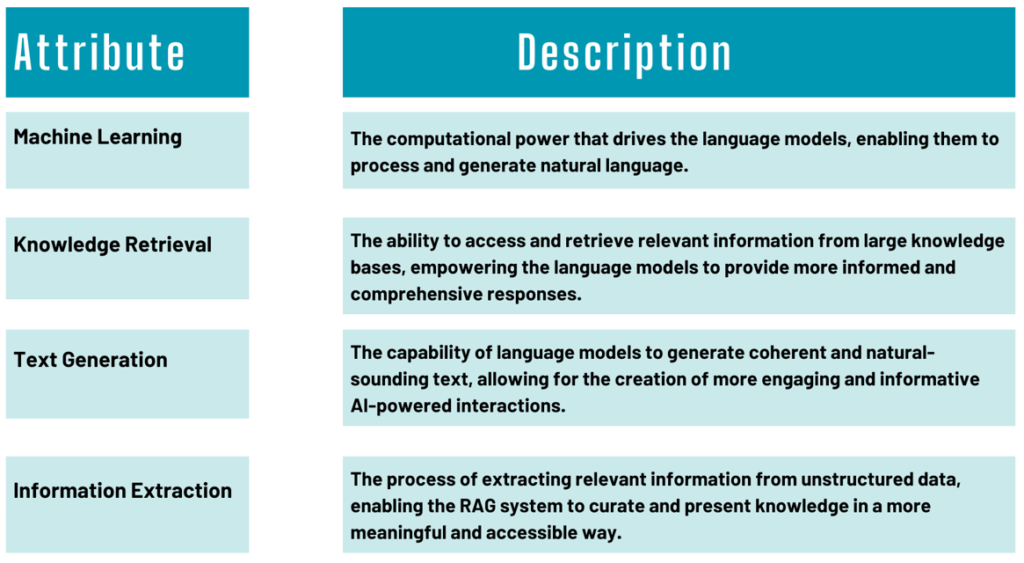
Building a RAG Model: A Step-by-Step Guide
Creating a retrieval-augmented generation (RAG) model is an exciting journey. It combines the strength of language models with knowledge retrieval systems. This new method has changed how we get and create information. It makes AI smarter and more context-aware.
Building a RAG model is a complicated method that combines multiple elements. Here’s a step-by-step instruction for comprehending this advanced technology:
- Selecting a Language Model: A powerful language model, such as GPT-3 or BERT, serves as the foundation for text production.
- Integrating the Knowledge Retrieval System: Retrieval-Augmented Generation models use a strong knowledge retrieval system. This adds important info to the language model. It might use a knowledge base or a document corpus.
- Training the Model: The model is fine-tuned to work well together. This ensures it does great in open-domain question answering and other tasks.
- Deploying the RAG Model: After training, the model is ready for use. It can help in many areas, like chatbots and information extraction.
To build a Retrieval-Augmented Generation model, you need to know a lot about machine learning, natural language processing, and info retrieval. Learning this can unlock the full power of retrieval-augmented generation. It changes how we interact with and find knowledge online.
Step | Description |
1. Language Model Selection | Choose a strong language model like GPT-3 or BERT as the base of the RAG model. |
2. Knowledge Retrieval Integration | Add a strong knowledge retrieval system, like a knowledge base or a document corpus, to give the language model the right info. |
3. Model Training | Make the language model and the knowledge retrieval system work together well. This improves the model’s performance in tasks like open-domain question answering. |
4. Model Deployment | Use the trained RAG model in different applications, such as chatbots, info extraction, and text generation tools. |
RAG for Beginners: Getting Started
Starting with Retrieval-Augmented Generation (RAG) can seem tough, especially if you’re new to AI and language models. This guide aims to make this complex tech easier to understand. It helps bridge the gap between AI and human understanding.
Unlocking the Potential of RAG
Retrieval-Augmented Generation is a new method that combines large language models (LLMs) with knowledge retrieval. This makes natural language processing tasks better. Unlike regular LLMs, RAG models can get info from outside sources. This leads to more accurate and relevant answers.
If you’re into making chatbots, automating info searches, or answering questions, Retrieval-Augmented Generation is key. Knowing the basics of RAG opens up new possibilities.
A Beginner’s Roadmap to RAG
- Start by learning what RAG is and how it’s different from traditional LLMs. Understand its benefits.
- Look into how Retrieval-Augmented Generation is used, like in answering questions or generating text.
- Find out how to make a RAG model. This includes picking a dataset, choosing an architecture, and fine-tuning.
- Know the limits and challenges of Retrieval-Augmented Generation models. Learn how to overcome them for better results.
- Keep up with the latest in Retrieval-Augmented Generation and large language models research.
By following this guide, you’ll get closer to using Retrieval-Augmented Generation effectively. It can change how we interact with and understand information.
“RAG has the potential to bridge the gap between AI and human understanding, making advanced language technologies more accessible and intuitive.”
The RAG Method: Revolutionizing Information Access
The Retrieval-Augmented Generation approach transforms how we obtain and process information. It combines language modeling and knowledge extraction. This improves the accuracy, contextualization, and informational value of responses. It is transforming areas such as open-domain question answering, text production, and conversational AI.
At its core, RAG uses large language models (LLMs) and knowledge retrieval. LLMs are great at creating natural language. Knowledge retrieval helps them understand and answer questions better. This combo makes Retrieval-Augmented Generation a powerful tool for getting relevant and detailed answers.
Retrieval-Augmented Generation excels in open-domain question answering. It can answer a wide range of questions accurately. By tapping into a vast knowledge base, RAG provides detailed and contextual answers that traditional models can’t match.
The Retrieval-Augmented Generation method also transforms text generation. AI systems can now create more coherent, factual, and engaging content. RAG-powered models can generate narratives and summaries that are real and context-specific.
In conversational AI, RAG shines too. AI assistants can have more natural and informative conversations. They address user queries with a deeper understanding, offering valuable insights that improve the user experience.
TheRetrieval-Augmented Generation method is a major step forward in AI. It bridges the gap between language models and knowledge retrieval. This opens up new possibilities for AI applications that truly understand and meet our needs.
Feature | Description |
Language Model | The language model component of a RAG system is responsible for generating natural language outputs, drawing on its deep understanding of language and patterns. |
Knowledge Retrieval | The knowledge retrieval component of RAG system is responsible for accessing and retrieving relevant information from a knowledge base or database, which is then used to enhance the language model’s outputs. |
Integration | The integration of the language model and knowledge retrieval components is a key aspect of the Retrieval-Augmented Generation method, allowing for seamless and contextual information access and generation. |
By using Retrieval-Augmented Generation, retrieval-augmented generation, knowledge retrieval, information access, and text generation, the RAG method is set to change how we interact with AI. It promises to revolutionize information access and extraction from AI systems.
“The Retrieval-Augmented Generation method represents a paradigm shift in how we approach information access and processing, seamlessly blending language models and knowledge retrieval to deliver unprecedented levels of understanding and responsiveness.”
RAG’s Impact on Large Language Models
Retrieval-Augmented Generation is critical to improving large language models (LLMs). It provides new ways to work with natural language and text. RAG enables LLMs to provide more accurate and relevant answers by utilizing external knowledge.
LLMs like GPT-3 and BERT have changed how we work with language. They can write like humans. But, they only know what they’ve been trained on. Retrieval-Augmented Generation lets them find and use new information, making their answers better.
By adding Retrieval-Augmented Generation to LLMs, they can use lots of different sources. This includes websites and academic papers. This helps them answer questions more fully and accurately.
This mix of language models and information extraction makes LLMs better at writing. They can find and use the right information. This makes their writing more accurate and interesting.
The future of AI is bright, thanks to Retrieval-Augmented Generation and LLMs. They will help in many areas, like talking to machines and creating content. This is just the beginning.
Limitations and Challenges of RAG LLMs
RAG, or Retrieval-Augmented Generation, is a big step forward in AI. It helps us find and use information better. But, it also has its own set of problems. We need to work on these issues to keep improving RAG.
Addressing the Shortcomings of AI-Powered Information Retrieval
One big worry with Retrieval-Augmented Generation is bias in the data it uses. If the data is biased, the model’s answers might show that bias too. We must make sure the data is good and fair.
Another problem is making Retrieval-Augmented Generation work with lots of data. As more data comes in, it gets harder to find what we need quickly. Scientists are looking for ways to make this process faster and better.
It is also difficult to combine RAG’s search and creation functions. We must ensure that the model makes good use of the data while avoiding text errors. This is an area where a lot of work is taking place.
Even with these challenges, Retrieval-Augmented Generation has a lot of promise. It could change how we get and use information. As we tackle these problems, we’ll see even more progress in AI and language.
Conclusion
Retrieval-Augmented Generation, or Retrieval-Augmented Generation, is changing how we get, process, and make information. It mixes language models with knowledge retrieval. This combo is making big waves in many areas, like answering questions and creating conversations.
RAG connects machine learning with human knowledge. It uses big language models and smart info retrieval. This lets AI systems dive into huge data pools, finding insights and giving answers that are both useful and fitting.
As AI keeps growing, Retrieval-Augmented Generation shows us the huge potential of mixing machine smarts with human knowledge. This mix boosts AI’s abilities and opens doors to a future where AI and humans collaborate. Together, they can explore new areas of understanding and discovery.