Table of Contents
Introduction
Did you know GANs can make images so real, even experts can’t tell they’re fake? This deep learning method is changing AI, leading to big steps in image making, language understanding, and more. In this article, we’ll dive into how GANs work, their huge potential, and the challenges ahead.
This is at the core of AI revolution: GANs They use a special training method where two models compete. This unsupervised learning lets it create diverse, realistic data. It’s opening doors to new AI innovations, from realistic images to better language understanding.
We’ll look into GANs’ basics, their place in deep learning, and their uses in India. We’ll meet the innovators and see how Generative adversarial networks are changing industries. This article aims to show you the full impact of GANs on AI.
Rise of Generative Adversarial Networks GANs
Generative adversarial networks a new concept in the field of artificial intelligence (AI), created a revolution in everything, including the way these artificial intelligence models have transformed the generation and learning from data.
Understanding the Need for Generative Models
Old AI models needed lots of labeled data. But, the real world has lots of data without labels. Generative models like Generative adversarial networks solve this problem. They make fake data that looks real.
The Pioneers Behind GANs
Renowned researchers like Ian Goodfellow and Yoshua Bengio started GANs. They have changed unsupervised learning with new adversarial training methods.
GANs work by competing neural networks. They can make very realistic and varied content. This has opened up new areas in AI, like computer vision and natural language processing.
The need for generative models is growing as data gets bigger. Generative adversarial networksare a big deal in AI. They could start a new era of innovation and discovery.
Demystifying GANs: How They Work
Generative adversarial networks are interesting models that change the game regarding generative modeling. The design is very intricate and has a rather smart training method, allowing for the creation of quite plausible and varied synthetic data.
The Generative adversarial networks architecture basically consists of two main constituents: the generator network and the discriminator network. They play a game wherein the generator creates fake data, and then the discriminator tries to spot it. This game makes the generator better at making the data look real.
This back-and-forth game helps the generator learn the patterns in the data. It can then make new data that looks just like the real thing. GANs are great for many tasks, like making images, adding data, finding odd data points, and even creating new ideas.
Understanding the Inner Workings of GANs
Let’s discuss the major parts of Generative adversarial networks and how they work together to give us a clearer picture of them.
- Generator Network: This artificial network creates fake data, such as images or text. It begins with noise which is then transformed into producing something realistic.
- Discriminator Network: This network tries to figure out if data is real or fake. It gives a score that shows how likely it is to be real.
- Adversarial Training. The generator tries to pretend to be as much a real data member as it can, whereas the discriminator tries to capture such pretenders. So this, in turn, strengthens the generation ability of a good data.
The ongoing battle between the generator and discriminator is critical to GAN performance. As they continue to play, the generator improves its ability to provide data that closely resembles the original.
Key Aspect | Description |
gans architecture | A two-network architecture consisting of a generator and a discriminator, engaged in a minimax game. |
generator network | Responsible for generating synthetic data, such as images, text, or audio. |
discriminator network | Tasked with distinguishing real data from the generated samples. |
adversarial training | The iterative process of training the generator and discriminator networks to improve their respective capabilities. |
minimax game | The constant competition between the generator and discriminator, driving them to become better at their tasks. |
GANs as : Supervised or unsupervised learning?
Generative adversarial networks created an a contentious discussion in the field of machine learning. Are they supervised learning , unsupervised learning, or something else? Let’s look at how GANs are influencing data production and model training.
The Blurring of Learning Paradigms
In the vast majority of instances of machine learning models, they are classified into two broad categories therefore the first category is supervised and the second one is unsupervised. Supervised learning deals with data that has been labelled in advance while finding the patterns in the data that has not been labelled before is described as unsupervised learning.
Still, Generative adversarial networks are unpredictable. They have a generator and a discriminator that participate in a game. The generator attempts to create fake data that appears authentic, while the discriminator attempts to detect the fakes.
This game between the generator and discriminator makes GANs hard to categorize. They learn to create new data without labels, but they also get feedback from the discriminator.
This change is big. Generative adversarial networks have made semi-supervised learning possible. They use a little labeled data and a lot of unlabeled data to train models. This has worked well in many areas, like making fake images and understanding language.
Also, Generative adversarial networks show the power of generative models. They can learn the data’s underlying patterns and make new, realistic samples. This is different from discriminative models, which focus on specific tasks.
As long as machine learning develops, GANs will remain a big thing. They are applied in those gaps between supervised and unsupervised learning.
The Power of Transformational GANs
Generative adversarial networks are transforming artificial intelligence. They now serve as grounds for realistic images and improved data. They can produce differentiated images that almost appear lifelike and open computer vision to new possibilities.
Image Synthesis and Data Augmentation
Image Synthesis and Data Augmentation GANs can create very realistic images. This has huge implications for image generation. It can develop special datasets that can be used for lots of tasks, such as training AI or augmenting present datasets with more data by applying data augmentation.
GANs really come into their own in image-to-image translation. They can easily translate images from one style to another. This gives artists and designers new avenues with style transfer
Technique | Description | Applications |
Image Generation | GANs can create highly realistic synthetic images from scratch. | Training computer vision models, creating custom datasets, data augmentation |
Image-to-Image Translation | GANs can transform images from one visual domain to another. | Style transfer, photo-realistic rendering, image enhancement |
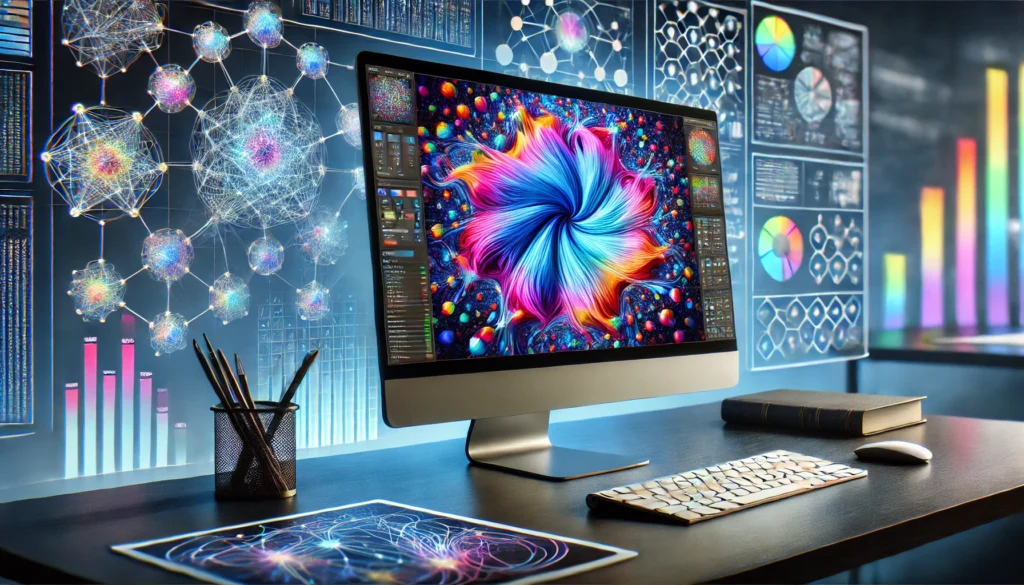
GANs in Natural Language Processing
Generative adversarial networks have progressed beyond simply creating images. They are currently making significant advances in natural language processing (NLP). These artificial intelligence models are utilized for a variety of linguistic tasks. This opens up new opportunities for creative writing and content creation.
GANs are great at text generation. They can create text that sounds real and makes sense. This is useful for chatbots and writing interesting content.
Generative adversarial networks also excel in language modeling. This means they can guess the next word in a sentence. They help make language models that are very good at many NLP tasks.
But, GANs in NLP have their own problems. One issue is “mode collapse,” where the model only uses a few outputs. Researchers are working hard to solve these problems.
As NLP grows, Generative adversarial networks will change how we create text and model language. They could make writing and talking to machines better. GANs in NLP are an exciting area in AI.
Task | Description | Example Applications |
Text Generation | Generating realistic and coherent text, mimicking human-like language patterns | Dialogue systems, content creation |
Language Modeling | Predicting the next word in a sequence, learning the underlying distribution of natural language | Machine translation, text summarization |
Dialogue Systems | Generating natural-sounding responses in conversational interfaces | Chatbots, virtual assistants |
Computer Vision: GANs’ Extraordinary Impact
Generative adversarial networks have transformed computer vision. They enabled picture synthesis, super-resolution, inpainting, and video creation. These models are excellent at processing and comprehending visual information.
Generative adversarial networks can make images that look very real. They do this by competing with a discriminator network. This competition helps them create images that are almost indistinguishable from real ones. This has improved image synthesis a lot.
GANs also excel in super-resolution. They can make low-resolution images look better. This is useful in many fields, like medicine and entertainment, where clear images are important.
Generative adversarial networks have also made a big difference in inpainting. They can fill in missing parts of images. This makes it hard to see where the original image was damaged.
But GANs’ impact doesn’t stop there. They can even create video sequences from scratch. This is a big deal for things like virtual reality and security.
Generative adversarial networks have really changed computer vision. They’ve opened up new possibilities for how we work with images and videos. The future looks even more exciting as we keep exploring what GANs can do.
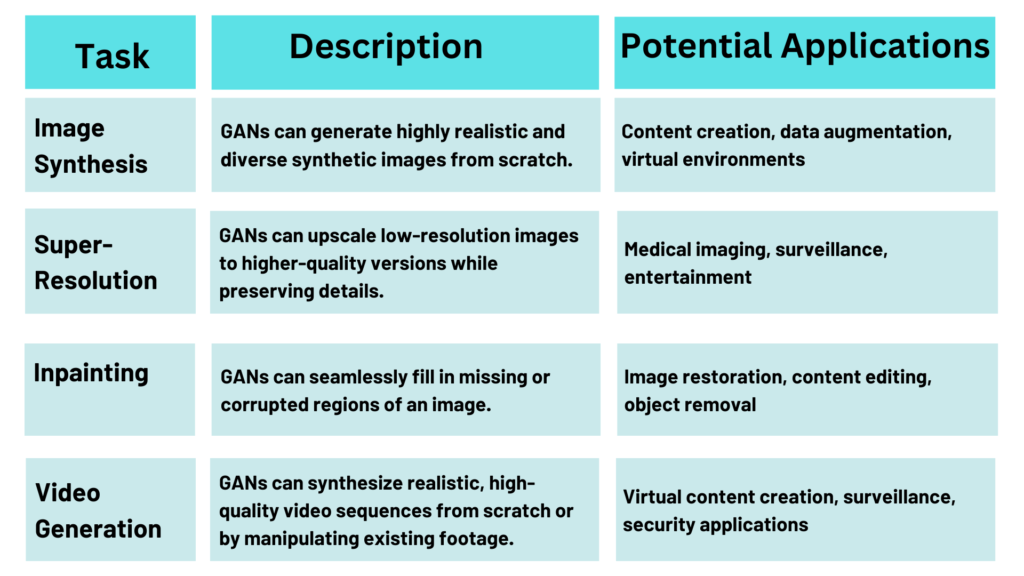
Anomaly Detection with GANs
Generative adversarial networks) are transforming artificial intelligence. They are no longer simply for making photos. They’re excellent at detecting patterns in noise and outliers in data.
Generative adversarial networks learn data patterns on their own, making them perfect for finding new things and oddballs. They learn from “normal” data. Then, they can spot data that’s different, marking it as an anomaly.
This skill is huge in many fields. It helps catch fraud in finance and check product quality in manufacturing. GANs help find problems early, saving time and money.
Identifying Patterns in Noise
Generative adversarial networks are good at finding odd data because they can handle complex data well. They have a generator and a discriminator that work together. This training helps them find small details that others might miss.
Approach | Description | Advantages |
---|---|---|
Anomaly Detection using GANs | Using GAN’s unsupervised learning capabilities to spot patterns in data and detect anomalies. | 1. Effective in high-dimensional, complex data 2. Adaptable to various domains and applications 3. Proactive approach to identifying issues |
The Creative Potential of GANs
Generative adversarial networks have opened up a new field in computational innovation. These AI models are altering the way we see art and creativity. They combine human and machine-generated material in novel ways. GANs are making AI art, generative art, and style transfer more interesting.
Generative adversarial networks work by having two neural networks compete. This unsupervised learning lets them create unique images. They learn from data to make original art that surprises us.
Unlocking the Potential of AI Art
Generative adversarial networks are making AI art that amazes us. They learn from lots of art to create stunning pieces. This could change how we make art, mixing human and machine ideas.
As ai art grows, we’ll see more amazing works. GANs can make everything from dreamy landscapes to abstract paintings. Their creativity knows no limits.
Challenges and Limitations of GANs
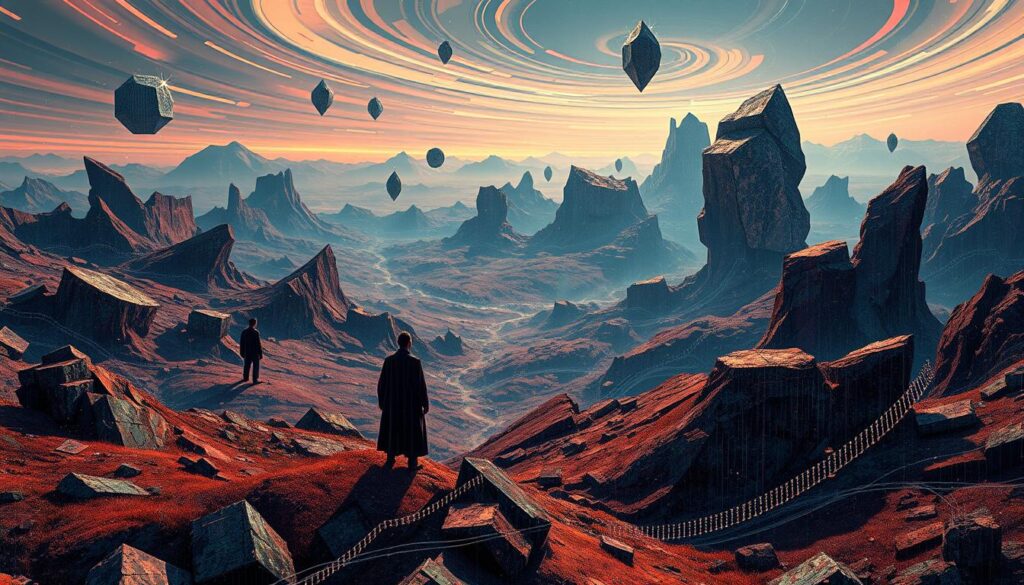
GANs (generative adversarial networks) hold significant potential in various sectors. Nonetheless, they encounter numerous obstacles and limits. Understanding these is critical to improving and advancing AI technology.
Mode Collapse and Other Pitfalls
One big problem with GANs is mode collapse. This happens when the generator only produces a few types of outputs. It fails to show the full range of what’s possible. Training instability is another issue, where the balance between the generator and discriminator can break down. This makes it hard for the networks to converge.
Vanishing gradients are also a challenge. These gradients get very small, slowing down training. Plus, Generative adversarial networks can be hard to understand, making it tough to fix problems. This lack of interpretability is a big concern.
Another issue is synthetic data bias. The data created by GANs might not truly reflect reality. This can lead to biased models and dangerous uses in the real world.
Challenge | Description |
---|---|
Mode Collapse | The generator network learns to produce a limited range of outputs, failing to capture the full diversity of the target distribution. |
Training Instability | The delicate balance between the generator and discriminator networks can be disrupted, leading to convergence problems. |
Vanishing Gradients | The gradients used to update the network parameters become extremely small, hindering the training process. |
Interpretability | The inner workings of Generative adversarial networks are not always clear, making it harder to detect and resolve faults. |
Synthetic Data Bias | The generated data may not precisely reflect the true underlying distribution, resulting in biased models and potentially damaging real-world applications. |
GANs: Beyond Image Generation
generative adversarial networks have grown beyond just making images. They are now changing many fields, like text, audio, and video. This shows how GANs are expanding into new areas.
Expanding Horizons: Text, Audio, and Video Generation
GANs can do more than just make pictures. They can also create moving and multi-modal content. Researchers have shown Generative adversarial networks can make text that makes sense and fits the context. This opens up new chances in natural language processing and content creation.
it can also make high-quality music, speech, and sound effects. This is changing how we make and enjoy audio.
GANs are even making videos that look real and smooth. This is great for virtual reality, movies, and games. It makes the experience feel more real.
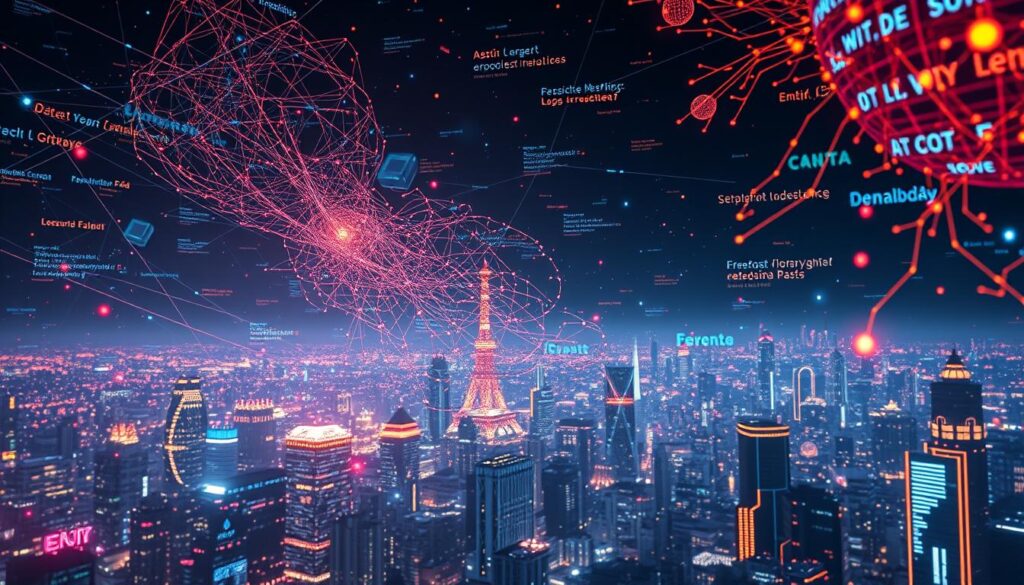
Integrating with Other AI Techniques
Generative adversarial networks work better when combined with other AI methods. Reinforcement learning-based GANs can learn to navigate and make content. Transfer learning-based GANs use what they’ve learned before to solve new problems.
This makes GANs even more useful in many fields. They’re changing how we create and research. The future of AI in making content is very promising.
Technique | Description | Applications |
---|---|---|
Text Generation | GANs may generate logical and relevant text, making them useful in natural language processing, content creation, and language translation. | • Automated content generation • Language translation • Conversational AI systems |
Audio Synthesis | GANs can generate high-quality music, speech, and sound effects, transforming the audio production landscape. | • Music composition • Voice generation • Sound design for media |
Video Generation | GANs can create realistic and seamless moving images, with potential applications in virtual reality, film production, and video game development. | • Virtual reality environments • Cinematic visual effects • Animated content creation |
Conclusion
Generative adversarial network have changed the game in artificial intelligence. They started as a new idea and now they’re amazing at many things. GANs have made machine learning, data creation, and even creativity better.
Generative adversarial networks can make fake data that looks real and varied. This has opened up new areas in fields like images, language, and finding odd data. They use a special training method to do things we never thought possible with AI. This has led to many new and exciting uses in different industries.
The future of GANs looks bright. They will keep getting better and finding new uses. In India and worldwide, GANs are making a big difference. They’re helping us innovate, make better choices, and explore new creative ways.