Table of Contents
Introduction
Think about a world in which machines can see, hear, and understand like we do. This is what deep learning promises. It’s a key part of artificial intelligence that’s changing how we use technology. Deep learning algorithms can now beat humans in tasks like image recognition and understanding language.
This breakthrough is opening doors in healthcare and making self-driving cars possible. It’s a big step forward.
Key Takeaways
- Deep learning would refer to the area of machine learning that applies artificial neural networks with the objective of simulating the human brain’s functionality while learning as well as making choices.
- Neural networks are the backbone of any deep learning system: they can recognize complex patterns inside large datasets.This lets machines do things better than humans.
- Deep learning beats traditional machine learning in areas like understanding language, seeing images, and predicting outcomes.
- Transformers, a type of deep learning, have changed how we understand and create language. They use self-attention mechanisms.
- Deep learning is leading to new ideas in many fields. It’s set to make a big difference in our lives soon.
In this tutorial, we will try to unravel big changes brought to artificial intelligence through deep learning. We’ll break down the basics of neural networks, what deep learning is not typically associated with, and its algorithms that are very powerful.
We’ll see how deeplearning is used in many areas, like understanding language and seeing images. We’ll also talk about its role in solving big problems and driving new ideas.
Understanding Deep Learning
Deep learning is a key part of machine learning that has changed AI a lot. It uses artificial neural networks to learn from data better than old methods. This makes it more efficient and smart.
What is deep learning?
Deep learning processes data using multiple layers of artificial neural networks. These networks function similarly to the human brain, assisting people in learning and making decisions based on complex information. this models can improve at tasks by learning from data in layers.
Fundamentals of Neural Network
Deep learning is fundamentally built on artificial neural networks. They have nodes, or “neurons,” that link in layers. These neurons receive data, apply math to it, and then send it to the next layer.
As data flows across the network, the connections between neurons shift. This allows the model to learn and improve at tasks. The network comprises three layers: input, hidden, and output. This configuration enables deep learning to handle challenging issues and discover valuable insights in large datasets.
this is changed many industries, including computer vision and speech recognition. It represents a huge step forward for AI. Deep learning is going to expand the capabilities of artificial intelligence as it advances.
Deep Learning VS Machine Learning
Deep learning and machine learning are sometimes mistaken with one another. But they are not exactly the same. Deeplearning is a more advanced form of machine learning. It is capable of handling complex data more efficiently and effectively.
Machine learning needs humans to pick out important data points. Deep learning, on the other hand, uses complex neural networks to find these points automatically. This makes it better at dealing with things like images and audio.
Deeplearning also does better with big data sets. It can find patterns that traditional methods miss. This makes it a key tool for many AI tasks, from seeing pictures to understanding language.
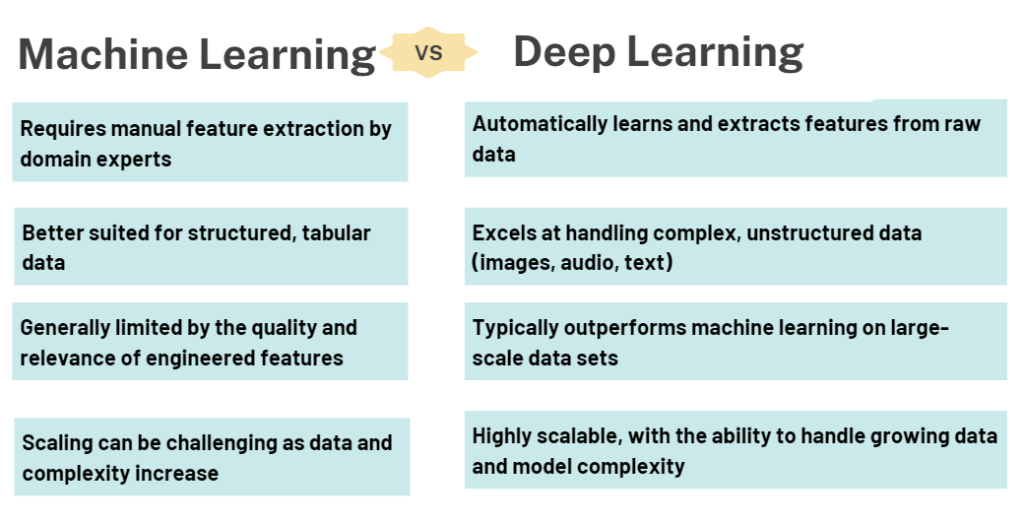
The Power of Neural Networks
Strong neural networks are used in deep learning. They are similar to the structure and function of the human mind. Two basic networks that would improve AI and machine learning developments are the Multilayer Perceptrons, known as MLP, and the Convolutional Neural Networks, or CNN.
Multilayer Perceptrons
Multilayer perception are artificial neural networks where the information is passed from an input layer to the output layer(or final result). This network can be learnt to associate complex data and thus finds most applications in classifications and regressions.
They handle very high-dimensional inputs with great efficiency. This attribute makes them a crucial tool in deep learning applications.
Convolutional Neural Networks
Convolutional Neural Networks (CNNs) are specifically designed for grid-like data, such as images, by utilizing local connectivity to efficiently extract features. The area for a CNN is most suitable for work in computer vision in the areas of image and object detection.
These neural networks have been significant in the progress of deeplearning and artificial intelligence. They opened new vistas for both machine learning and computer vision.
The true power of neural networks lies in their ability to learn and adapt, allowing them to tackle complex problems that were once considered intractable.
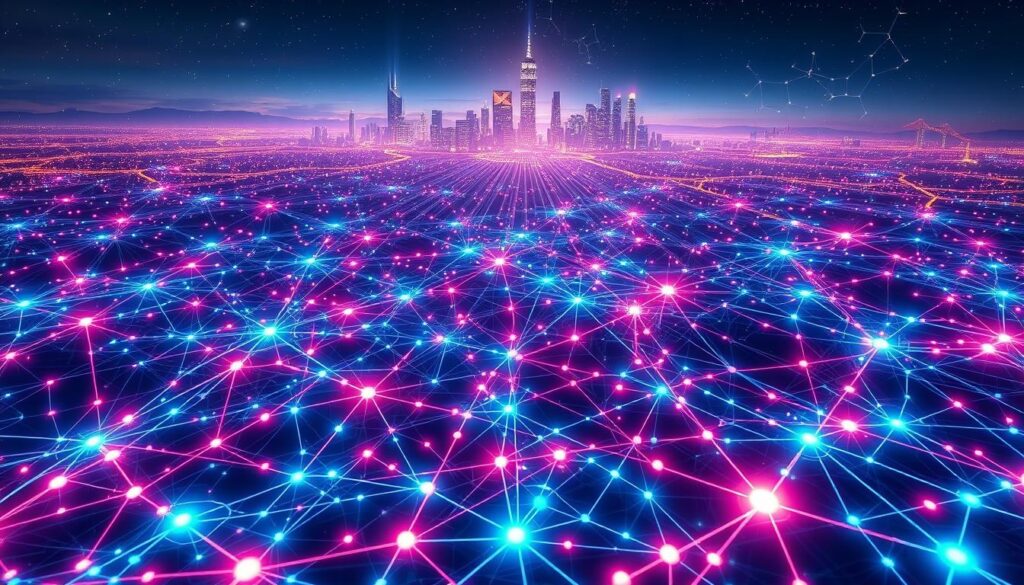
Deep learning algorithms.
it is the basic aspect of machine learning and artificial intelligence. It becomes the new frontier of transforming many industries. At its heart are complex algorithms that power deep learning.
One of the key algorithms in deeplearning is backpropagation. It enables the adjustment of model parameters to improve performance. Another important algorithm that improves the model is gradient descent, which changes the model’s parameters in order to minimize error. Activation functions such as sigmoid, ReLU, and tanh are of significant importance. They enable the model to learn complex concepts by introducing non-linearity.
- Backpropagation: Enables efficient adjustment of neural network parameters to the smallest error possible.
- Gradient Descent: It optimizes the model by adjusting parameters iteratively to reduce a loss function.
- Activation Functions: Introduce non-linearity to the model, so as to let it learn complex patterns.
These algorithms enable models to do many things-they can understand language and see images. Using these algorithms, we can do more with deeplearning.
How Transformers Work in Deep Learning
Transformers have changed the game in deeplearning, especially in natural language processing. They use attention mechanisms to better understand complex language. This makes them more effective than older deeplearning models.
Attention Mechanisms
At the core of transformers are attention mechanisms. These allow the model to focus on key parts of the input. Unlike older models, transformers can look at the whole input at once. This helps them catch important relationships and dependencies.
Self-Attention and Transformer Architecture
Transformers go further with self-attention. They learn to pay attention to different parts of their own input. This self-aware approach helps them grasp the input deeply. As a result, they excel in tasks like machine translation and text summarization.
The transformer architecture has transformed deep learning, especially for natural language tasks. Thanks to attention, transformers have raised the bar for language understanding and generation. They’re opening doors to even more advanced uses of deep learning in the future
The Importance of Deep Learning
it is more than just a tech upgrade. It’s changing how we solve problems and innovate. This AI method helps us tackle tough challenges, find new discoveries, and explore what’s possible with machine learning.
At the core of deep learning is the neural network. It’s like a brain in tech, with layers that mimic the human brain. By training these networks on lots of data, we can spot patterns, predict outcomes, and gain insights we couldn’t before.
Deeplearning excels at learning from data in layers. It can find features and meanings in raw data. This skill is key in areas like computer vision, language processing, and predictive analytics, where deep learning shines.
“Deep learning is part of the future of artificial intelligence, but already it is beginning to impact the world that we live in.”
It will come to play crucial creativity in deep learning and solve the fundamental issues in all the different spheres. New openings are being created from the Health, Finance sectors thus transforming the potential avenues in artificial intelligence and machine learning applications. Deep learning can unlock full capacity to innovate and solve problems. Thus, it will lead to a future we can always push the limits of what’s possible.
Deep Learning Applications
it is a subset of artificial intelligence machine learning(AIML). It has transformed industries and changed how we connect with our surroundings. this encompasses natural language processing and computer vision, making our interactions almost completely natural and intuitive.
Natural Language Processing
Now, deep learning has opened up a great field in natural language processing. Great accuracies have been achieved both in understanding human languages and producing them as machines can do so much better today than ever in human history. This is due to deep neural networks.
Deep neural networks work marvelously on translation, text composition, and emotion analysis. They further enable us to talk much more naturally with machines.
Computer Vision
Deeplearning has also changed computer vision. Algorithms like convolutional neural networks can understand images like humans do. They’re used in many areas, from healthcare to self-driving cars.
These systems can recognize images, find objects, and even understand the meaning of images. This is making a big difference in many industries.
Application | Deep Learning Capabilities |
Natural Language Processing | Machine translation, text generation, sentiment analysis |
Computer Vision | Image recognition, object detection, semantic segmentation |
Deep Learning in Predictive Analytics
The field of predictive analytics is changing fast, thanks to deeplearning. This advanced machine learning can find new insights in complex data. It’s changing how companies predict trends, spot patterns, and make smart decisions.
Deep learning models can find important features in data that others miss. They use neural networks to handle big, complex data. This means they can make more accurate predictions and forecasts.
Deeplearning is also great at cognitive computing. It works like the human brain to solve complex tasks, like understanding language and images. This is helping many industries, like healthcare and finance, use their data better.
Deeplearning is also good at mining data and recognizing patterns. It uses special techniques to find hidden connections in data. This helps make forecasts more precise and insightful.
As more companies adopt deeplearning, they will be able to make better forecasts and judgments. This gives them a major advantage over their opponents. Deeplearning is creating new opportunities for organizations to solve challenges and innovate
Attribute | Deep Learning | Traditional Machine Learning |
Feature Extraction | Automated feature extraction from raw data | Requires manual feature engineering |
Model Complexity | Highly complex, multi-layered neural networks | Relatively simpler models (e.g., linear regression, decision trees) |
Performance on Large Datasets | Excels at handling large, high-dimensional datasets | May struggle with large, complex datasets |
Adaptability | Highly adaptable and can learn from new data | Often requires retraining with new data |
Benefits of Deep Learning
- Deeplearning is a vital application of artificial intelligence. It allows solving complicated problems in several fields. It proves to be much more effective than traditional machine learning; it therefore does bring about a change in the manner of data analysis and problem-solving approaches.
- Deep learning is good at dealing with large volumes of complex datasets. Deep neural networks are capable of processing much information. They manage to find patterns and connections that older methods cannot.
- It thus makes deep learning excellent for image recognition, natural language processing, and so much more. It works great for predictive analytics which entails quite a lot of huge complex data.
- it is also superb at several tasks as compared to traditional methods. The capability has proven to be better than human beings at recognizing images. This has brought very tremendous upgrades in diagnosing diseases in hospitals, fitting intelligent cars, and surveillance.
- The main benefit of deeplearning is its capability to scale up. Deep learning models can scale in accordance with the increase in data size and complexity, which makes the models more correct and reliable over time.
- This adaptability and future-proofing give deep learning a solution that always appears better with improvements in technology.
- Deeplearning has also changed the way we think about problems and make decisions. It reveals hidden insights from data. This in turn has enabled business organizations to make smarter decisions by making more-informed choices. It brings better efficiency, cost savings, and success.
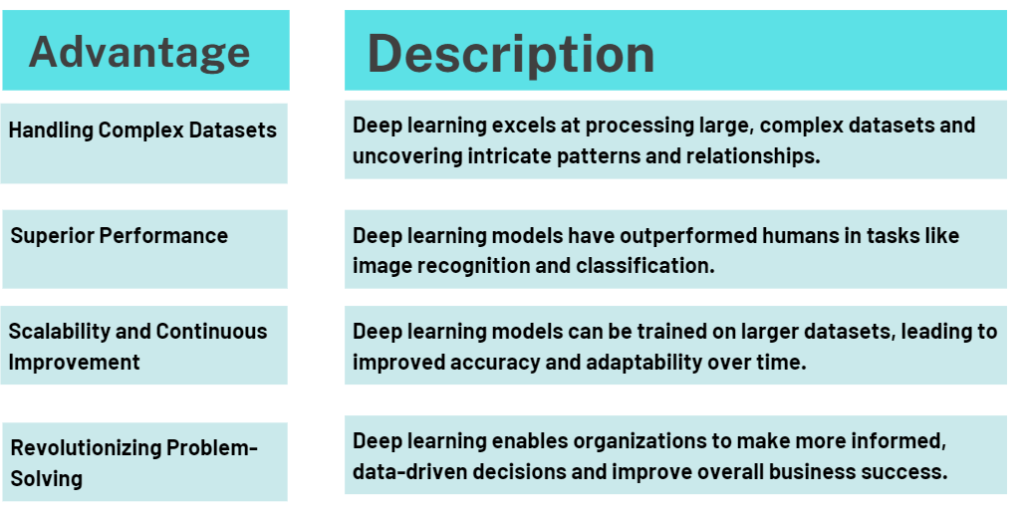
Conclusion
In this detailed look, I explored how deeplearning is changing artificial intelligence. We covered the basics of neural networks and the exciting uses of deeplearning. I showed you the main ideas, methods, and new developments that are making this tech grow.
Looking to the future, deeplearning will keep changing how we solve problems and find new things. Its huge potential is exciting, and I’m eager to see how it will change our world.
Deep learning has already shown its value in many areas, like understanding language and seeing images. It’s also been improved by working with transformers and attention mechanisms. This has made solving tough tasks and finding new ideas easier.